AI Strategy for the DACH Region: Achieving a Competitive Advantage in 8 Steps
Artificial Intelligence (AI) has long since moved beyond the realm of futuristic scenarios to become a tangible driver of innovation and efficiency within companies. Particularly in the economically significant DACH region, an increasing number of organizations are recognizing the transformative potential of this technology.
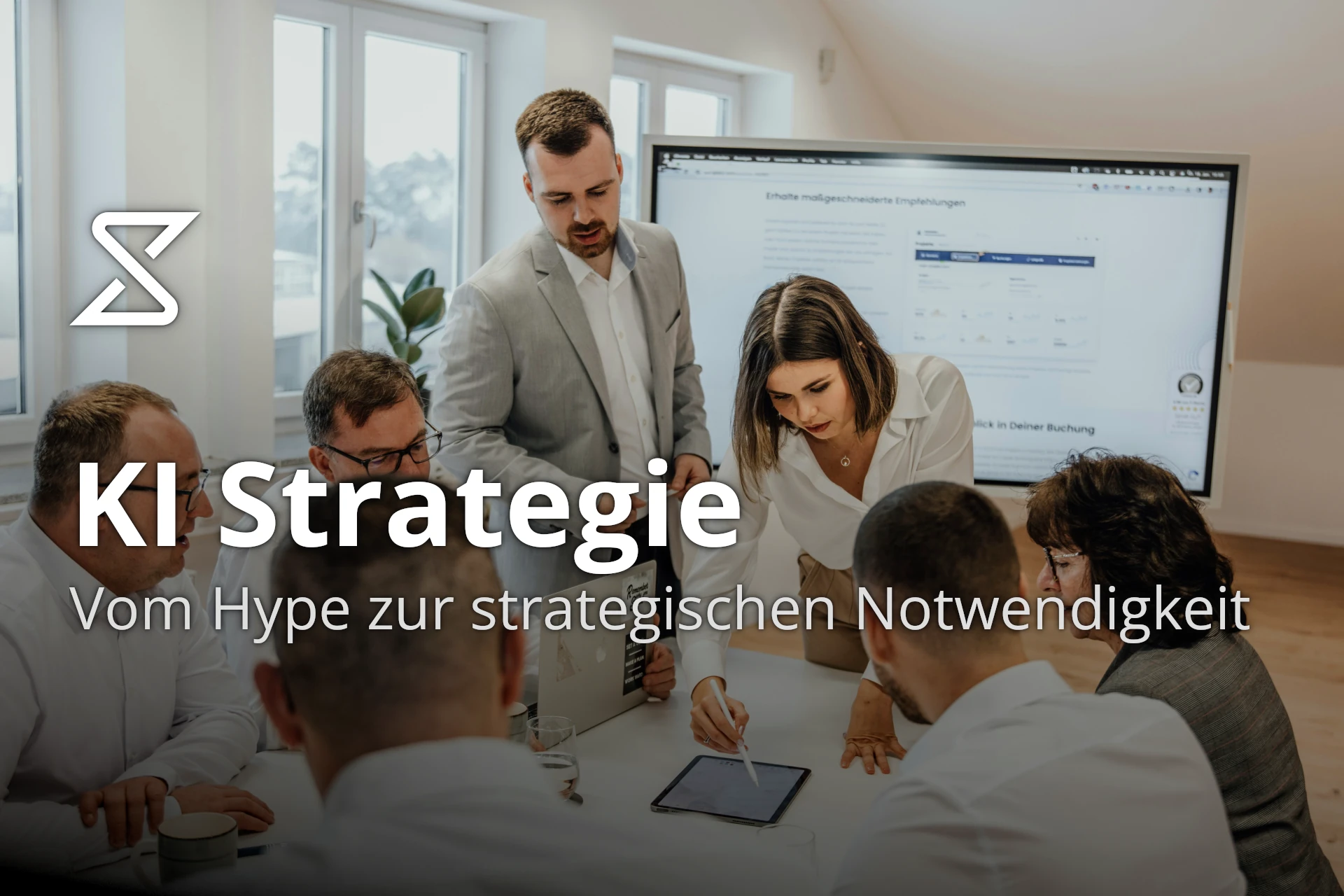
AI in the DACH Region – From Hype to Strategic Imperative
In 2025, AI remains a persistent topic, accompanied daily by new tools and applications. Yet, amidst this rapid development, a crucial question arises for businesses: How can the full potential of AI be strategically leveraged to secure a sustainable competitive advantage?
The Current AI Landscape in the DACH Region: Between Advancement and Hesitation
AI adoption in the DACH region is progressing, yet it presents a mixed picture. In Germany, for instance, more than half (57 percent) of companies are actively engaged with AI, and one in five (20 percent) are already using it – a significant increase.1 Globally, over 70 percent of organizations have implemented AI in at least one business function.2 Key drivers include improved AI accessibility (43 percent), pressure to reduce costs (42 percent), and competition (31 percent).3 Generative AI, such as ChatGPT, is used by 40 percent of Germans.1
Investment readiness is growing: global AI spending was estimated at 500 billion US dollars for 2024.2 In Switzerland, investments in AI startups doubled in 2024.4 The potential is enormous: for Switzerland alone, the annual GDP contribution from generative AI is estimated at 80-85 billion CHF (+11 percent).5 Nevertheless, there is still reluctance: only about one-third of German companies plan concrete AI investments in 2024.6 Per capita funding for B2B AI startups in the DACH region is ten times lower than in the US, indicating a less mature ecosystem.7
More critical, however, is the gap in strategic maturity. A Cisco study (2023) revealed that only 7 percent of Swiss companies are considered “pioneers” in AI implementation (vs. 14 percent globally).8 A Hays survey (2024) confirmed this: only one-third of DACH organizations have a defined AI strategy.8 Globally, 18 percent of companies lack any strategy at all.3 Often, there is a shortage of metrics for success measurement and long-term financing plans. This discrepancy is also evident regionally: Swiss companies are the most confident (58 percent see potential, 57 percent expect it to be a top investment priority), while managers in Germany (34 percent) and Austria (35 percent) are more skeptical regarding ROI and implementation capabilities.9
The Urgency of a Strategy: Risks of Non-Adaptation
Hesitation carries risks. Companies without a clear AI plan could be overtaken by more agile competitors. Nearly half of German firms (48 percent) believe that companies without AI have no future.1 Ignoring the disruptive power of AI can quickly erode competitiveness.
Operational hurdles are also present: lack of data quality/availability (56 percent)3, integration difficulties, skills shortages, scaling problems10, high costs, regulatory hurdles3, and inadequate change management (64 percent)11 are the biggest challenges.
Without a strategy, inefficiencies from isolated solutions and the “pilot trap” also loom: promising pilots fail during company-wide rollout. Only about half (54 percent) of AI pilot projects make it to production.3 A five-year delay in utilizing generative AI could reduce the additional GDP potential in Switzerland from 11 percent to 3 percent.9
Outlook: Competitive Advantages Through a Targeted AI Strategy
It is clear: a structured, strategic approach is essential. A well-thought-out AI strategy acts as a compass, creates clarity, increases efficiency, opens up new business areas, improves decisions, enables personalized customer experiences, and ultimately secures a decisive competitive advantage in the DACH region.
The Unbeatable Advantages of a Well-Conceived AI Strategy for DACH Companies
A clear AI strategy is a fundamental investment in future viability and offers numerous benefits:
Clarity and Orientation
It sets the “North Star” for all AI activities, creates a common understanding of goals and measures, clarifies roles and responsibilities, and gives employees and external stakeholders security and confidence. Resources are used purposefully.
- Example (Industry): A German machine manufacturer defines clear goals for predictive maintenance10 and AI-supported quality control12, giving teams direction and facilitating budget allocations.
Increased Efficiency and Productivity Gains
AI automates routine tasks, reduces costs, and accelerates processes (e.g., 50% faster response times in customer service). More importantly, employees can concentrate on more complex, creative tasks (seen as an advantage by 72% of executives). Efficiency improvement is often a primary goal.8
-
Example (Industry): A Swiss precision manufacturer uses computer vision for quality control, reducing errors and inspection times.12
-
Example (Retail): M-Preis in Austria increases productivity and reduces task load by over 60 percent with AI-supported task management.13
Tapping into New Business Opportunities
AI is a catalyst for innovation, enabling new, data-driven products, services, and business models.7
-
Example (Finance): A Swiss bank14 uses AI for personalized investment recommendations, differentiating itself and creating new revenue streams.15
-
Example (Manufacturing): A German automotive supplier offers Predictive Maintenance as a Service, transforming its business model.10
Informed Decisions Through Data Analysis
AI analyzes vast amounts of data, recognizes patterns, and creates forecasts that exceed human capabilities.16 A strategy ensures that this capability is systematically used for better, data-driven decisions.
-
Example (Retail): Rossmann uses an ML model for optimal planning of self-checkout Kiosks.13
-
Example (Logistics): A logistics company dynamically optimizes routes using AI, saving fuel and time.2
Personalized Customer Experiences
AI enables a deep understanding of customers through data analysis, leading to tailored offers and communication. This increases customer satisfaction and loyalty.17 65 percent of consumers are open to AI improving their experiences.3
-
Example (Insurance): Allianz uses AI for proactive customer warnings (e.g., floods) and personalized products.18
-
Example (E-commerce): An online optician like Mister Spex provides personalized recommendations in real-time.19
Competitive Advantage Through Innovation
Strategic AI deployment positions companies as innovators.16 They can react faster and differentiate themselves from the competition.
- Example (Healthcare): An Austrian medical technology company develops AI for more precise analysis of medical images.4
Avoiding the “Pilot Trap” and Siloed Solutions
A comprehensive strategy prevents promising pilots from failing due to a lack of coordination or resources (“Pilot Trap”).7 Only about half of pilots reach production readiness. A strategy ensures systematic evaluation and scaling of successful approaches, avoids isolated siloed solutions, and unlocks the full value potential of AI across the enterprise.
Your Roadmap: In 8 Detailed Steps to a Tailored AI Strategy in the DACH Region
Developing a successful AI strategy requires a systematic approach. The following eight steps offer guidance for companies in the DACH region:
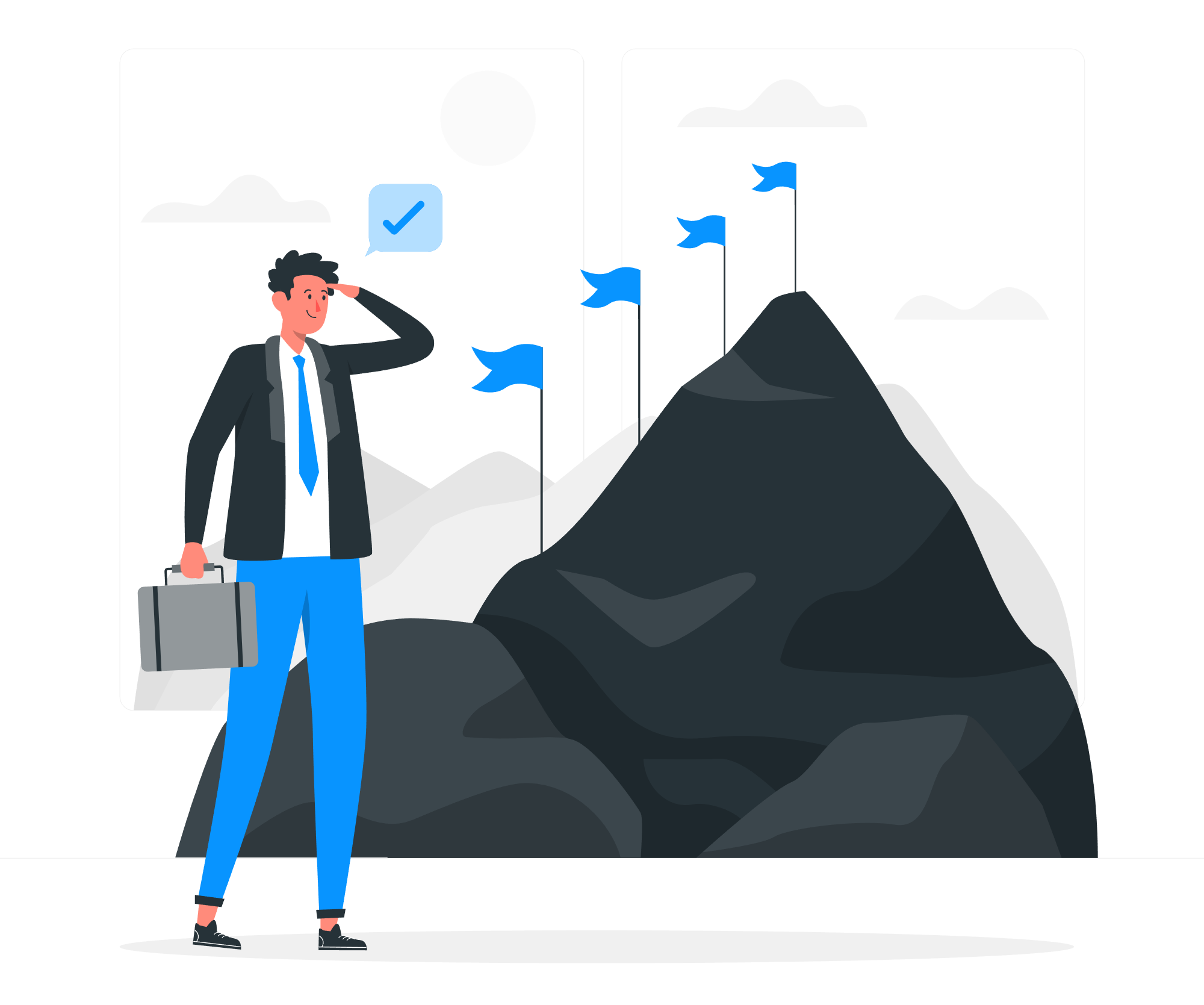
Step 1: Clarify Overarching Goals
The AI strategy must build upon the corporate strategy.20 Define concrete, measurable goals (e.g., increase market share, enhance efficiency) that AI is intended to support. Use methods such as SWOT analyses for AI, an adapted Balanced Scorecard, or OKRs (Objectives and Key Results) to specify goals and make them measurable. Consider industry-specific priorities in the DACH region.
Step 2: Allocate Resources and Know-How
Success requires the right resources. Assemble an interdisciplinary core team (technical, business departments, IT, legal, change management) with clear roles. An AI coordinator or an “AI Center of Excellence” can be beneficial. Address the skills shortage through internal training16, external expertise21, and leveraging ecosystems.22 Develop a long-term financing plan covering technology, personnel, data, implementation, and maintenance.
Step 3: Analyze Challenges and Potentials (Use Case Identification & Evaluation)
Identify concrete use cases with the greatest added value. Combine three approaches20:
- Problem-oriented: Where are the biggest pain points or unsolved customer needs?
- Technology-oriented: What opportunities do core AI capabilities (image recognition, language processing, etc.) offer your business?
- Data-oriented approach: What insights and applications can be derived from your existing data?
Use workshops, competitive analyses, and best practices for idea generation. Consider industry-specific relevance in the DACH region (see table below for examples).
Industry | Typical AI Use Cases (Examples) |
---|---|
Industry / Manufacturing (Industry 4.0) | Predictive Maintenance, Automated Quality Control, Process Optimization, Supply Chain Optimization |
Finance / Banking / Insurance | Fraud Detection, Personalized Advice, Risk Management, Process Automation, Chatbots |
Retail / E-commerce | Personalized Recommendations, Dynamic Pricing, Demand Forecasting, Automated Checkouts, Chatbots |
Healthcare / Pharma | Medical Image Analysis, Personalized Therapy Plans, Hospital Management Optimization, Drug Discovery |
Automotive | Autonomous Driving, Predictive Maintenance, Production Optimization, Intelligent Assistance Systems |
Other (Cross-Industry) | Marketing & Sales, HR, IT Security, Logistics |
Table: AI Use Cases: Examples from leading DACH industries
Step 4: Establish Ethical Guidelines and Regulatory Framework (Ethics & Compliance)
Responsible AI must align with European values23 and be human-centric. Trust, transparency, and fairness are central. Risks such as bias, data protection violations, or manipulation must be minimized.24
For companies in the DACH region, two regulatory frameworks are of utmost importance: the EU AI Act and the General Data Protection Regulation (GDPR). A detailed analysis of the impact of the EU AI Act on companies can be found in a separate blog post.
Develop your own guidelines beyond legal requirements. Orient yourself with frameworks (NIST25, OECD25, EU Commission26, IEEE24). Establish internal governance (ethics committees). Integrate ethics into development and procurement processes (bias mitigation, explainability (XAI), privacy by design, audits). Compliance and ethics are strategic imperatives, minimize risks27, and strengthen trust.
Step 5: Clarify Data Availability and Infrastructure (Data & Technology)
A solid foundation of high-quality data and powerful technology is crucial, but often a bottleneck.
Data Governance & Data Quality:
“Garbage In, Garbage Out” particularly applies to AI.28 Poor data quality is a top challenge. Robust data governance is essential29: clear goals and responsibilities, data standardization30, quality management and measurement, data cleansing, traceability (data lineage), access control, and lifecycle management. Ensure that relevant, representative, and high-quality data is available for training and operation.31 Break down data silos.8
Technical Infrastructure:
AI requires computing power (often GPUs/TPUs)8, scalable storage (cloud storage)32, high-performance networks (high bandwidth, low latency)33, and the right software stack (frameworks like TensorFlow, PyTorch).34
Cloud vs. On-Premise:
Companies face the choice of operating their AI infrastructure in the cloud, on their own servers (on-premise), or in a hybrid model:
- Cloud: Flexible, scalable, pay-as-you-go.35 Offers AI services and MLOps platforms. Challenges: latency, security/compliance (GDPR), cost control.
- On-Premise: Maximum control, potentially better for security/data protection.36 High initial investments, effort for maintenance/scaling.
- Hybrid: Combines advantages, requires orchestration.34
The choice depends on the company’s specific requirements, use cases, budget, and security and compliance needs.
MLOps (Machine Learning Operations):
Transfers DevOps principles to ML32 to automate and standardize the model lifecycle (data collection, training, validation, deployment, monitoring). Key practices: automated pipelines, CI/CD, versioning, Infrastructure as Code (IaC), monitoring. MLOps platforms (MLflow, Kubeflow) accelerate development, improve quality, and enable scaling. Investments in data, technology, and MLOps are crucial to move beyond the experimental stage.
Step 6: Evaluate Prioritization and Feasibility (Project Prioritization)
Systematically evaluate and prioritize identified use cases. Select projects with the greatest strategic value and acceptable risk/effort. An effective tool is the Value vs. Feasibility Matrix (also known as Impact vs. Effort Matrix). This framework helps to assess and visually classify projects based on two central dimensions:
- Axis 1: Economic Added Value (Value / Impact): Contribution to company goals (revenue, costs, customer satisfaction, quality, risk, differentiation37).38
- Axis 2: Feasibility (Feasibility / Effort): Technical (data, algorithms, infrastructure), organizational (know-how, processes, culture, budget), and external (market, partners, regulation) implementability.39
The positioning in the matrix provides recommendations for action:
- High Value, High Feasibility (Priority 1 - Implement / Quick Wins): These projects offer the greatest leverage and should be tackled with high priority and moved into the testing or pilot phase. They deliver quickly visible successes and build momentum. Example: Upgrading existing software to improve performance.40
- High Value, Low Feasibility (Priority 2 - Postpone / Major Projects): These projects are strategically important, but their implementation is complex, resource-intensive, or fraught with high uncertainties. They require careful planning and should possibly only be tackled once necessary prerequisites (e.g., data availability, know-how development) have been established or quick wins have been successfully implemented. Example: Developing a completely new, groundbreaking AI technology.40
- Low Value, High Feasibility (Priority 2 - Limit / Fill-ins): These projects are easy to implement but have only a limited strategic impact. They should only be pursued if resources are available and they might support other, more strategic projects or fill gaps. Example: Performing routine maintenance tasks with AI support.40
- Low Value, Low Feasibility (Priority 3 - Avoid / Thankless Tasks): These projects offer little benefit while involving high effort and risk. They should generally not be pursued further. Example: Implementing an insignificant feature that ties up disproportionate resources.40
In addition to the value-feasibility assessment, other factors can influence prioritization, such as a detailed risk assessment (financial, operational, reputational), the scalability of the solution beyond the initial use case39, and the strategic urgency of the project.
It is important to understand that prioritization is not a one-time static act. The framework conditions change: technologies evolve, new data sources are tapped, internal know-how grows, regulatory requirements change. Therefore, projects currently classified as “low feasibility” should not be entirely discarded but regularly re-evaluated. An AI strategy should include a mechanism for dynamically adjusting the use case portfolio.
Step 7: Develop and Test Prototypes (Agile Prototyping & MVP)
Validate prioritized projects through prototypes and Minimum Viable Products (MVPs) before full implementation. An agile, iterative approach is valuable given AI uncertainties.41
Agile Methods: Short development cycles (sprints), functional increments, close collaboration, continuous feedback, adaptability.42
Minimum Viable Product (MVP)43: The simplest version of an AI solution that delivers core value and allows maximum learning with minimal effort. Purpose: test hypotheses, validate assumptions, gather user feedback, minimize risk, shorten time-to-market. Process: problem definition, target group focus, feature prioritization (core value only), development & testing, feedback collection, iteration (Build-Measure-Learn).
Prototyping: Simple prototypes (mock-ups) before the MVP help visualize concepts and gather very early feedback. Involve users early on.44 The MVP approach is an important risk management tool and should be standard practice.
Step 8: Communicate Strategy and Scale Initiatives (Scaling & Change Management)
The real value of AI comes from scaling and embedding it within the company. This requires communication, change management, training, and success measurement.
Communication and Expectation Management: Clearly and transparently communicate the strategy, goals, and progress. Set realistic expectations to avoid resistance.11
Change Management for AI Implementation: AI implementation is disruptive. Professional change management is critical (up to 47% higher goal achievement). Inadequate change management is a top challenge (64%)11. Strategies: impact assessment, stakeholder alignment, resistance management (addressing fears), participation, clear responsibilities.
Employee Training & Upskilling: Close skill gaps through targeted training. Use modern learning formats (interest among 64% of employees21). Establish a learning culture.
Scaling: Systematically transfer successful pilots to other areas. Adapt infrastructure and processes. Develop a clear scaling strategy to overcome the “pilot trap.”
Success Measurement & ROI: Demonstrate the value of AI investments.45 Use a structured methodology (e.g., ROI Methodology®):
- Define goals and KPIs (linked to Step 1).
- Establish a baseline.
- Record costs (development, operation, personnel, etc.).
- Measure benefits (quantitative: cost savings, increased revenue, etc.; qualitative: brand image, employee satisfaction).
- Calculate ROI (ROI = (Net Benefit / Costs) * 100%). NPV approach for long-term consideration.
- Analyze and Adjust: Report results, optimize strategy.
Scaling is an organizational transformation. Change management and success measurement are crucial for sustainable competitive advantages.
Conclusion: Shape Your AI Future in the DACH Region – Strategically and Successfully
AI holds enormous potential for companies in the DACH region. However, the key lies not in experimentation, but in a well-thought-out, company-wide AI strategy. Challenges such as data quality, skills shortages, regulation (EU AI Act, GDPR), and change management are real but surmountable.
The 8-step process presented offers a structured roadmap. Now is the time for companies in Germany, Austria, and Switzerland to embrace AI as a strategic lever. Those who hesitate risk falling behind. Those who act proactively secure sustainable competitive advantages.
Start Your AI Journey with Solon Labs
Developing and implementing a comprehensive AI strategy is complex and requires in-depth know-how – from use case identification and technology assessment to compliance (GDPR & EU AI Act), technical implementation, and scaling.
Solon Labs is your specialized partner for tailor-made AI solutions in the DACH region. We understand the local conditions and will accompany you through each of the 8 steps. We help you avoid pitfalls like the “pilot trap” or compliance risks and unlock the full potential of AI for your competitive advantage. Our focus on innovation and legal certainty gives you the necessary security.
Take the first step now:
- Schedule an appointment for a non-binding initial consultation: Let’s analyze your specific challenges, potentials, and strategic goals in the field of AI together. Our experts will give you an initial assessment and show possible ways forward.
- Participate in a customized workshop: In an interactive workshop with our experts, develop the cornerstones of your individual AI strategy, perfectly tailored to the needs and goals of your company.
Contact Solon Labs today and shape your successful AI future in the DACH region with us!
Footnotes
-
Erstmals beschäftigt sich mehr als die Hälfte der Unternehmen mit KI | Presseinformation | Bitkom e. V., Source ↩ ↩2 ↩3
-
Key Statistics Driving AI Adoption in 2024 - HyperSense Software, Source ↩ ↩2 ↩3
-
AI Adoption Statistics 2024: All Figures & Facts to Know - Vention, Source ↩ ↩2 ↩3 ↩4 ↩5 ↩6
-
EY Startup Barometer: Investitionen in KI-Startups verdoppeln sich | News - investrends.ch, Source ↩ ↩2
-
Wo stehen Unternehmen bei der Implementierung von KI …, Source ↩ ↩2 ↩3 ↩4 ↩5
-
EY European AI Barometer 2024 | EY - Deutschland, Source ↩ ↩2
-
Entwicklung von KI-Lösungen für Unternehmen - Vention, Source ↩ ↩2 ↩3
-
The Hackett Group: Report Reveals GBS AI Adoption Accelerating, With 63% Seeing Early Gains - Stock Titan, Source ↩ ↩2 ↩3
-
Produktion - IT-Consulting in Frankfurt am Main - Innovaforge, Source ↩ ↩2
-
Vom Hype in die Filiale: KI kommt im Einzelhandel an - Retail …, Source ↩ ↩2
-
Zeit der Experimente bei Schweizer Banken ist vorbei - Finews, Source ↩
-
Datengetriebenes Private Banking durch künstliche Intelligenz - Zuehlke, Source ↩
-
KI am Arbeitsplatz: Ihr Wettbewerbsvorteil fürs Jahr 2025 | AlfaPeople DE, Source ↩ ↩2 ↩3
-
35 powerful customer service statistics for 2024 - ServiceNow, Source ↩
-
Einsatz von KI im Handel: Wie Künstliche Intelligenz den Handel revolutioniert, Source ↩
-
KI-Toolbox für Versorgungsunternehmen: Modul Use Cases - Fraunhofer IAO - KODIS, Source ↩ ↩2
-
The Lord Of The Indexes: The AI Readiness Index - SwissCognitive, Source ↩
-
Artificial Intelligence Strategy of the Austrian Federal Government - Digital Skills and Jobs Platform, Source ↩
-
What is AI Governance? Principles & Examples Explained - University of San Diego Online Degrees, Source ↩ ↩2
-
AI Governance Frameworks: Guide to Ethical AI Implementation - Consilien, Source ↩ ↩2
-
Top 9 AI Data Governance Best Practices for Security, Compliance, and Quality, Source ↩
-
KI-Compliance: Best Practices für das Trainieren von KI mit First-Party-Daten - DataGuard, Source ↩
-
How Data Governance Improves Data Quality: Concepts and Best Practices - Dataddo Blog, Source ↩
-
2024 Government AI Readiness Index - Oxford Insights, Source ↩
-
Accelerating AI and ML Projects with DevOps and MLOps: Best Practices for Data Scientists, Source ↩ ↩2
-
Building the Right Foundation: Key Infrastructure for MLOps Platforms - Rafay, Source ↩ ↩2
-
KI im Dachdeckerhandwerk – Chancen nutzen, Zukunft gestalten, Source ↩
-
Project Prioritization: 5 Steps to Identify ROI-Driven Opportunities - edison365, Source ↩
-
Bewertung und Priorisierung von KI-Use Cases - WiWiEn, Source ↩
-
How to Evaluate and Prioritize AI Projects: A Value-Feasibility Framework for Maximum Impact | Elementera, Source ↩ ↩2
-
Project Prioritization Matrix [Free download], Source ↩ ↩2 ↩3 ↩4
-
MVP in Agile | Tips for Success with Minimal Viable Products - Miro, Source ↩
-
Minimum Viable Product in Agile: Benefits Explained - NEKLO, Source ↩
-
Minimum Viable Product (MVP): What is it & Why it Matters - Atlassian, Source ↩
-
6 Change Management Strategies To Avoid Enterprise AI Adoption Pitfalls - Gigster, Source ↩
Frequently Asked Questions
What is the current status of AI adoption in companies in Germany, Austria, and Switzerland?
What are the biggest challenges in introducing AI in companies?
- Lack of data quality and availability
- Difficulties in integrating AI with existing IT systems
- Shortage of qualified specialists
- Problems with scaling pilot projects ("Pilot Trap")
- High costs
- Regulatory hurdles (e.g., EU AI Act, GDPR)
- Process complexity and unrealistic expectations
- Insufficient change management